Mohn Technology is developing several autonomous underwater vehicles. One of them is an automatic net inspection drone for the aquaculture industry. The project is partially funded by FHF and will reduce the risk of escaped salmon by inspecting the facility in a safe, efficient and environmentally friendly way.
We have done most of the AUV (Autonomous Underwater Vehicle) in a computer simulation environment to quickly get a overview of how the AUV reacts to different scenarios, with wave motion, water current etc.
Since we do a lot of work in a simulation environment, we are able to transfer the navigation algorithms to all compatible underwater vehicles without too much extra work. In the field trial we used an existing prototype used for pelagic fisheries research, because it had a suitable stereo camera, IMU (Inertia Measurement Unit), depth sensor and onboard computer.
The umbilical is only for communication and manual override as the vehicle is battery powered. The finished product will be truly autonomous without any cable. This is done in order to reduce the risk of entanglement in a crowded net pen with numerous obstacles like ropes, sensors, cables and cleaner fish housing.
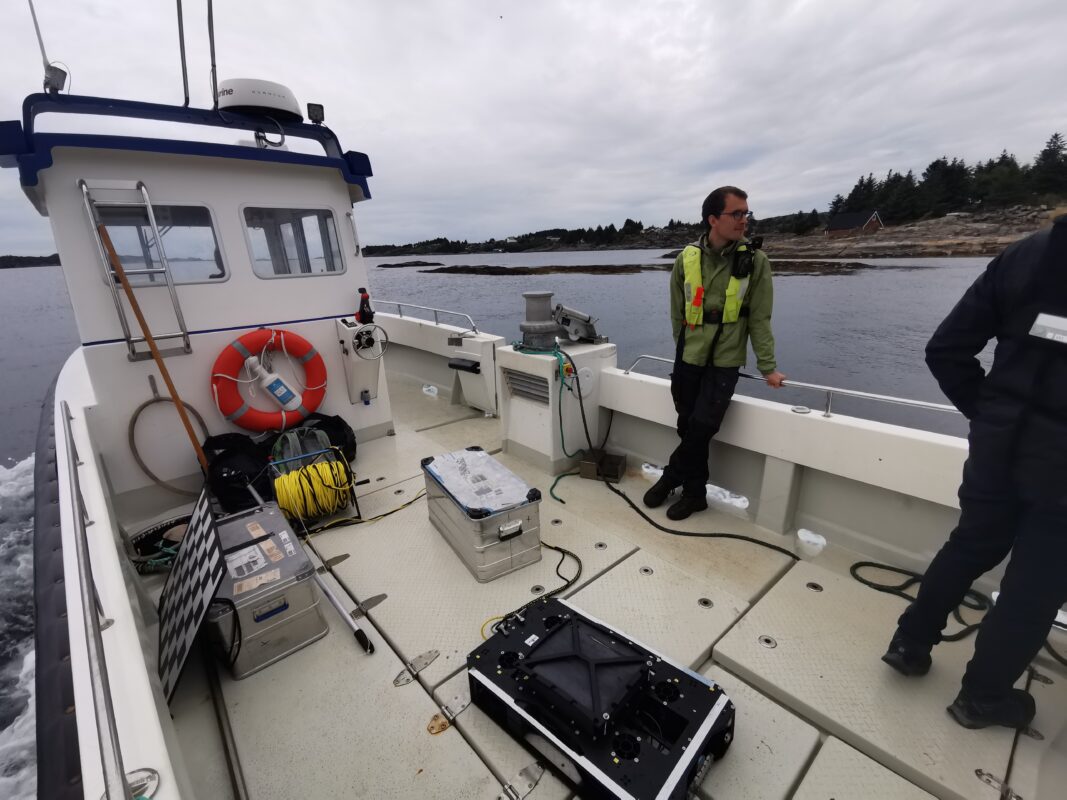
The trail was performed at an operational aquaculture facility of a Norwegian fish farmer at the west coast. Even though the facility was pretty exposed, the weather was fair and the sea was calm.
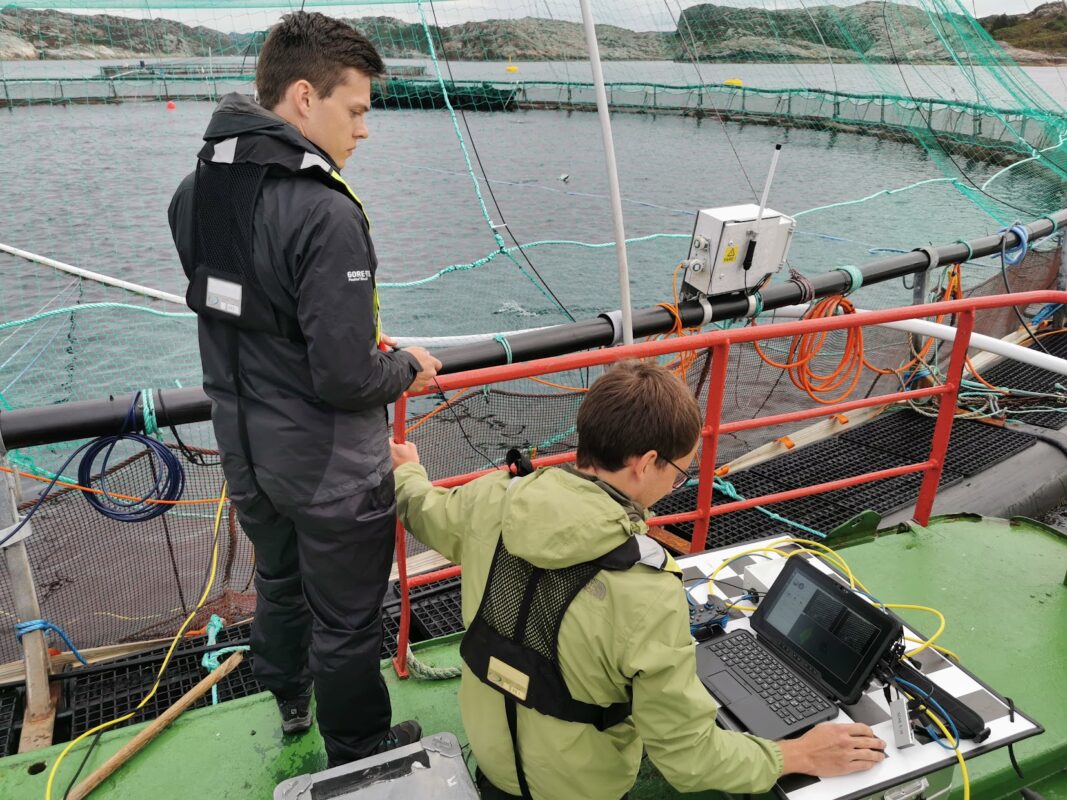
The net tracking algorithms worked well, and the test provided vital experience with real life operations in an active fish farm. We were impressed how well the algorithms manged to filter out disturbances like salmon swimming between the vehicle and the net. The salmon seemed to be very little affected by the drone, and calmly swam in close proximity to the vehicle.
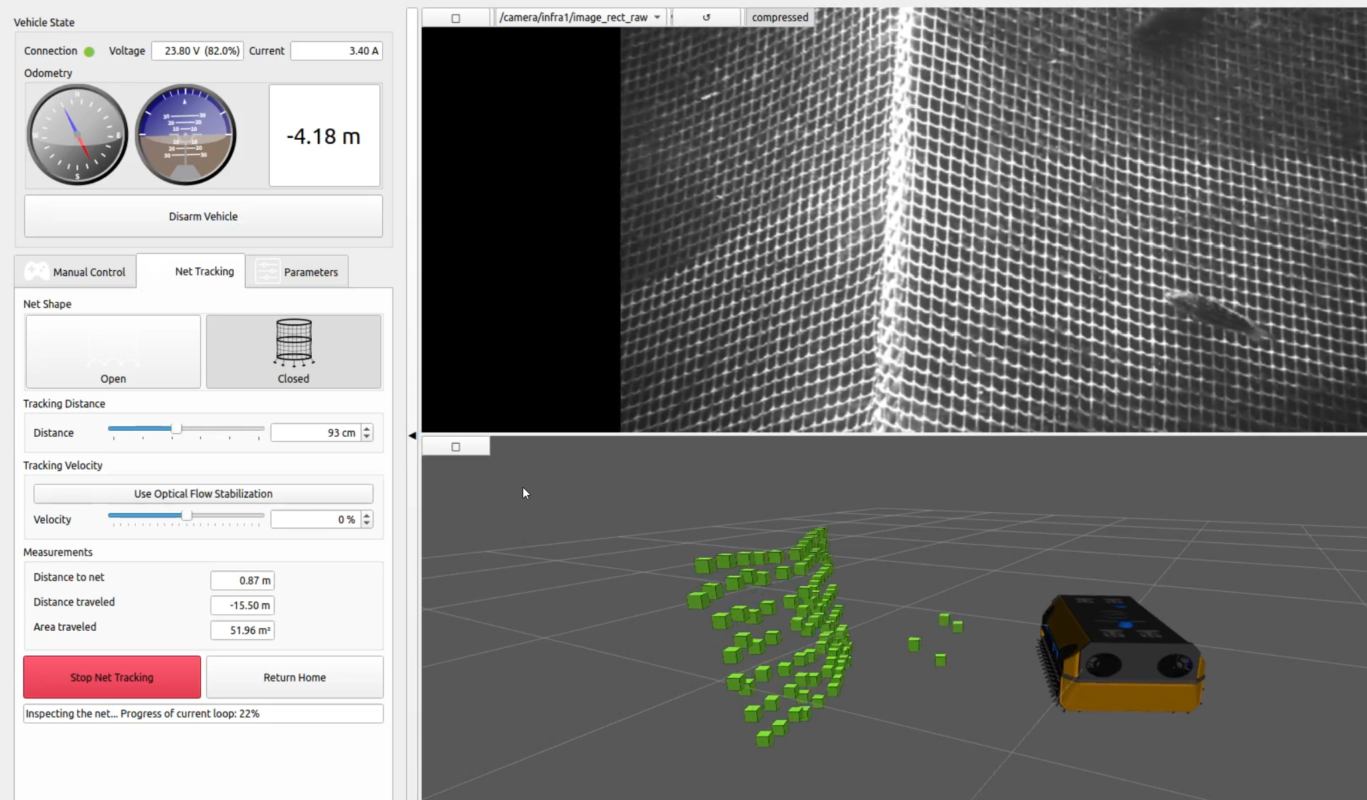
Image above shows the current GUI that allows for changing distance to net and velocity during the autonomous operation. The lower visualisation shows the drone position relative to the net. The green blocks are 3D positions of the net generated from the stereo camera imagery.
As we expected, the natural state of a fish farm is more than just a orderly net. There are stitches, reinforcement ropes, equipment on the outside, algea and seaweed and fish that will interfere with a machine vision algorithm and produce false positives. This has lead us to work with AI (Artificial Intelligence) based machine vision in conjunction with a more conventional machine vision. We believe this approach will make a robust and efficient automatic inspection tool.
We are really pleased with the development progress and are exited to continue the work.